Towards Scalable Neural Representation for Diverse Videos CVPR 2023
Video
Abstract
Implicit neural representations (INR) have gained increasing attention in representing 3D scenes and images, and have been recently applied to encode videos (e.g., NeRV, E-NeRV). While achieving promising results, existing INR-based methods are limited to encoding a handful of short videos (e.g., seven 5-second videos in the UVG dataset) with redundant visual content, leading to a model design that fits individual video frames independently and is not efficiently scalable to a large number of diverse videos. This paper focuses on developing neural representations for a more practical setup -- encoding long and/or a large number of videos with diverse visual content. We first show that instead of dividing videos into small subsets and encoding them with separate models, encoding long and diverse videos jointly with a unified model achieves better compression results. Based on this observation, we propose D-NeRV, a novel neural representation framework designed to encode diverse videos by (i) decoupling clip-specific visual content from motion information, (ii) introducing temporal reasoning into the implicit neural network, and (iii) employing the task-oriented flow as intermediate output to reduce spatial redundancies. Our new model largely surpasses NeRV and traditional video compression techniques on UCF101 and UVG datasets on the video compression task. Moreover, when used as an efficient data-loader, D-NeRV achieves 3%-10% higher accuracy than NeRV on action recognition tasks on the UCF101 dataset under the same compression ratios.
NeRV vs. D-NeRV
Comparison of D-NeRV and NeRV when representing diverse videos. NeRV optimizes representation to every video independently while D-NeRV encodes all videos by a shared model and contioned by keyframes from each video.
Model overview
D-NeRV takes in key-frame pairs of each video clip along with all the frame indices and outputs a whole video clip at a time. The decoder block predicts the flow estimation to warp the visual content feature from the encoder, then fuses the visual content by the spatially-adaptive fusion module and finally models temporal relationship by the global temporal MLP module.
- The Visual Content Encoder captures clip-specific visual content.
- The Flow Preidction module estimates task-oriented flow to reduce spatial redundacies across frames.
- The Spatially-adaptive Fusion (SAF) module utilizes the content feature map as a modulation for decoder features.
- The Global Temporal MLP (GTMLP) module exploit explicit temporal relationship of videos.
Video Compression
UVG Dataset
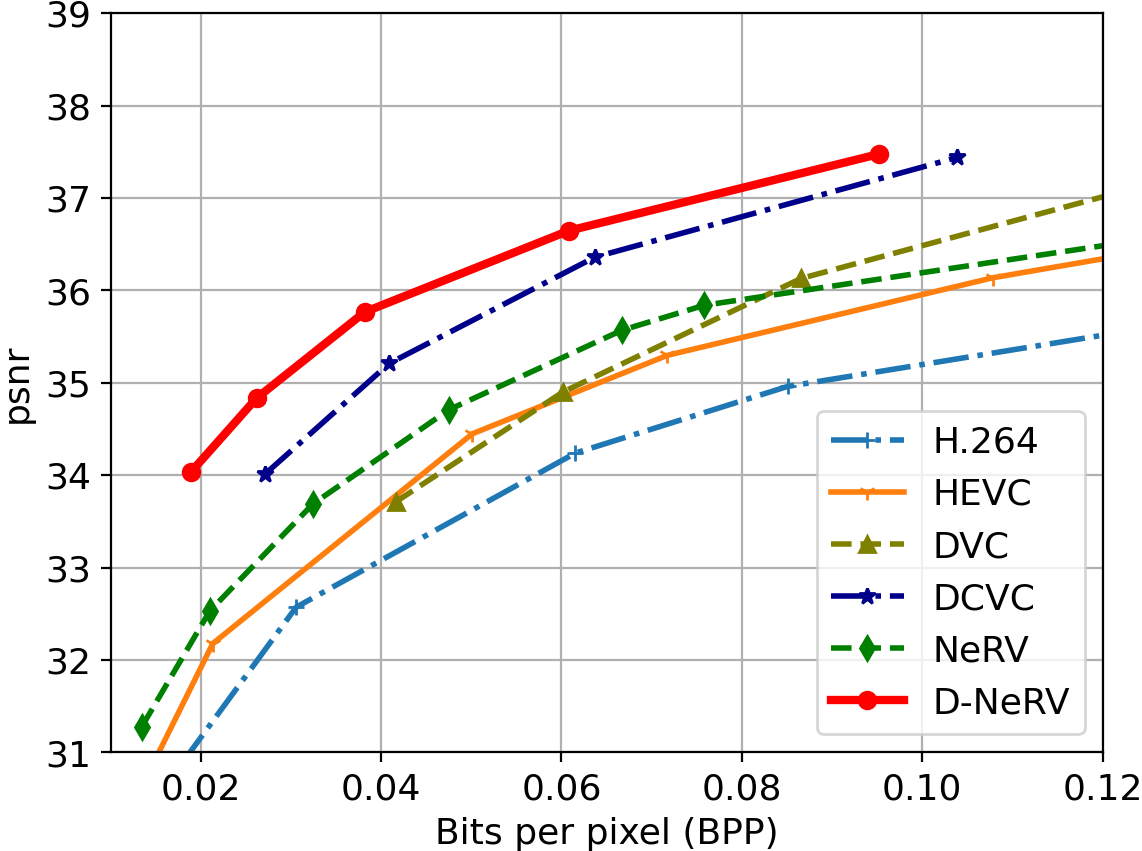
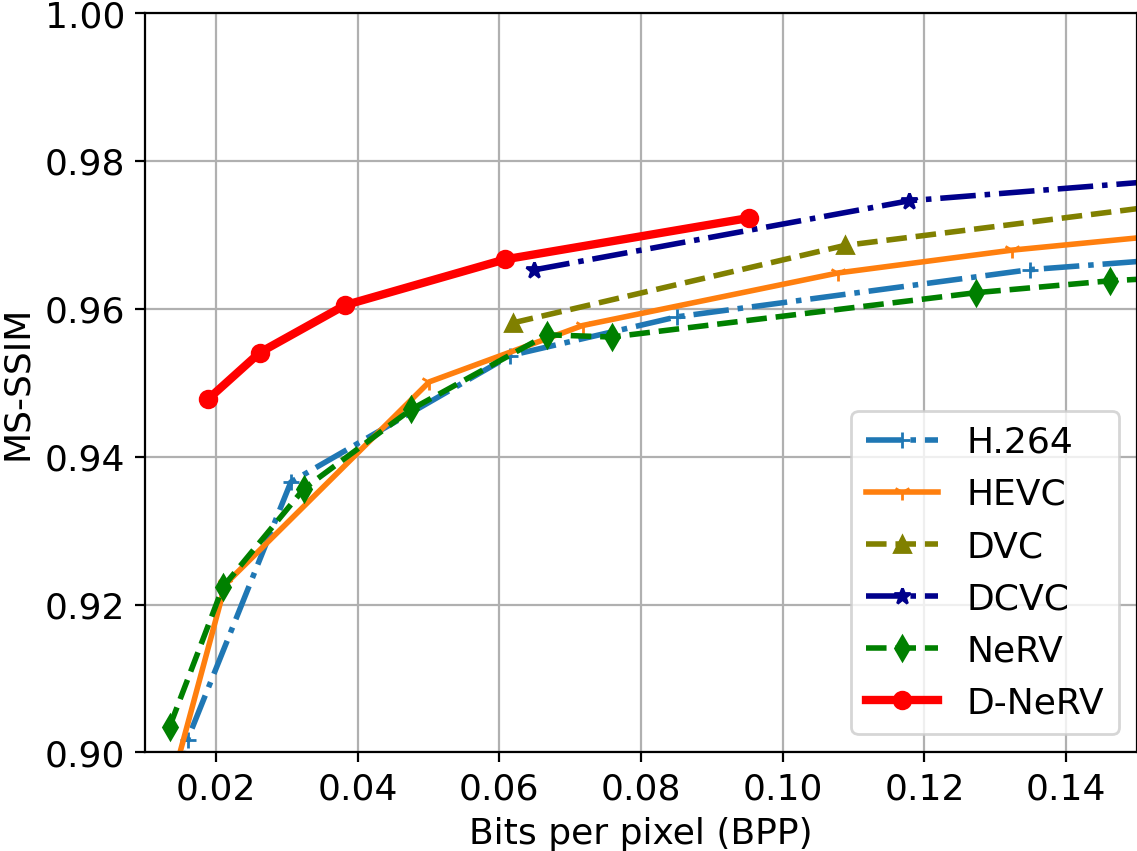
UCF-101 Dataset
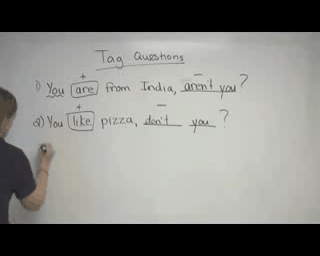
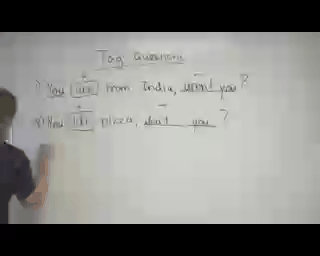
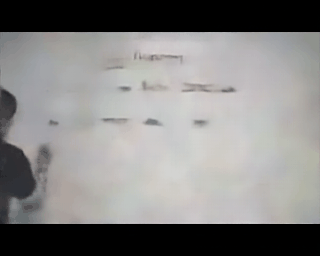
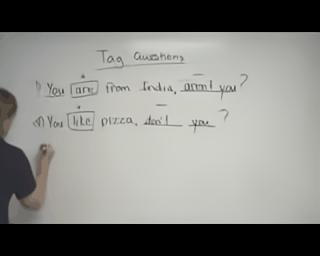
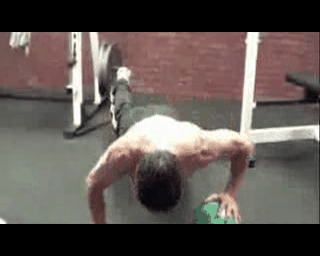
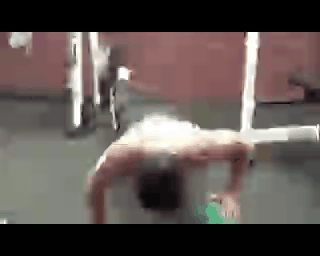
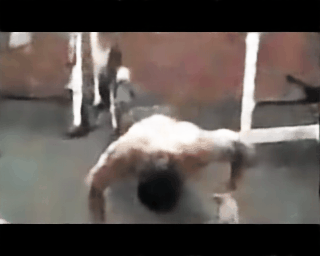
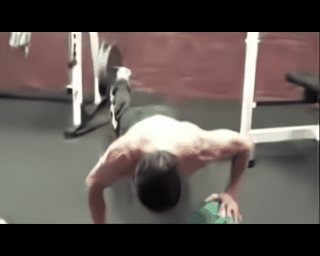
Comparision under the same compression ratio
Action Recongnition
UCF-101 Dataset
Video Inpainting
Citation
@inproceedings{he2023dnerv,
title = {Towards Scalable Neural Representation for Diverse Videos},
author = {He, Bo and Yang, Xitong and Wang, Hanyu and Wu, Zuxuan and Chen, Hao and Huang, Shuaiyi and Ren, Yixuan and Lim, Ser-Nam and Shrivastava, Abhinav},
booktitle = {Proceedings of the IEEE/CVF Conference on Computer Vision and Pattern Recognition (CVPR)},
year = {2023},
}
The website template was borrowed from Ben Mildenhall.